
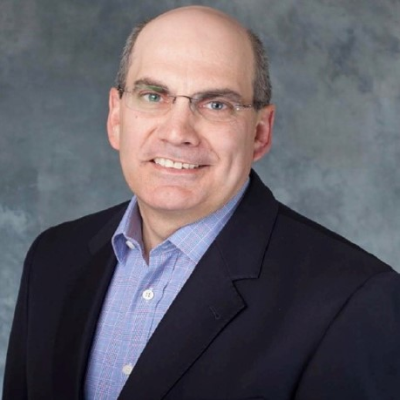
In our Ecosystem Explorer Series, we interview leaders from organizations who are advancing access to health data. Today’s interview is with Cliff Li, Senior Director of Consulting, and Bob Morrison, Vice President of Data Analytics at Clarivate.
Cliff Li is a Partner in Commercial Strategy and Market Access Team at Clarivate and is the lead of the US RWD service line. Cliff acts as a Subject Matter Expert on a wide range of issues related to US pricing, coverage, brand strategy, and market access dynamics. Cliff’s expertise in patient-longitudinal analytics and leveraging a wide range of data assets allows him to assist clients in executing more sophisticated engagements to help develop actionable, value-driven strategies and tactics. Cliff holds an MPH in Health Policy & Law.
Bob Morrison brings 30+ years of consulting, technical product development, and senior leadership experience in healthcare, technology, and digital marketing. He brings 12+ years of experience designing, building, and operating high-volume patient data integration workflows linking disparate patient data types for high-impact biopharma analytics and commercial monitoring. He has led the data workflow build, operation, and client support for Symphony Health, DRG, and Clarivate. He holds an MBA from the University of Rochester.
Clarivate™ is a leading global provider of transformative intelligence. Clarivate offers enriched data, insights & analytics, workflow solutions, and expert services in the areas of Academia & Government, Intellectual Property, andLife Sciences & Healthcare. Clarivate’s connected data, deep expertise, and intelligence platforms empowerLife Sciences and healthcare companies to deliver safe, effective, and commercially successful treatments to patients faster. Clarivate is home to Cortellis™, solutions for real-world data, medtech, market access and commercialization, and deep consulting expertise.
Introduction to Claims Data
Cliff and Bob, thank you for joining us today. Starting with the basics, how do you define claims data?
Cliff: Claims data is information that captures services that occurred at the point of care - whether that be at a pharmacy, hospital, medical office, or other place of service - and also captures how it was adjudicated by payers. The power of claims data is the granularity it provides on the patient, payer, and treating provider.
What are the differences between open and closed claims, and why would a researcher choose one versus the other?
Bob: Open claims data include both medical claims and pharmacy claims primarily sourced from clearinghouses, pharmacies, and software platforms. Since open claims encompass multiple data types, they offer insights into patient touchpoints across the healthcare landscape with no limitations on timeframe. They are payer agnostic, so patients will not be lost when switching insurance plans. The recency of the data is also a differentiator–many medical, pharmacy, and lab visits within open claims data can be seen as recently as yesterday, offering near-real-time reporting and tracking.
Closed claims data, on the other hand, are derived from the insurance provider (or payer) and capture nearly all events that occur during a patient’s enrollment period, including medical and pharmacy visits and transactions for both retail and specialty settings. This provides a valuable view into the patient journey and connects patients’ diagnoses, actions, and decisions along the way with minimal gaps.
Cliff: Open data can be leveraged in ways that closed data is most commonly used, such as health outcomes or healthcare resource utilization studies, through patient stability panels, which allow the user to benefit from the scope and scale of an open dataset but provide strict continuous enrollment criteria to “close” the patient sample.
Closed databases have some limitations. Closed claims data have at least a 90-day data lag and tend to be limited to specific enrolled populations, which means certain insurers or employers. This can lead to bias in analysis unless handled correctly. For instance, if a patient switches to a different healthcare plan, they may either disappear from the database or appear as a new patient in the new plan's database.
Another limitation of closed databases is that they do not provide any information about any supplementary insurance or services that may have been paid for by cash.
The Applications and Benefits of Working with Claims Data
With so many data types available to researchers, what makes claims data uniquely valuable to researchers? What are some common and emerging applications of de-identified claims data?
Clif: Longitudinal de-identified claims data is rich in granularity as it not only provides insight into a patient’s healthcare journey but also demographics related to the patient, demographics of the treating providers and the affiliated health systems, as well as the payer responsible for adjudicating and reimbursing claims. Other consumer industries may be touted for knowing their customer through data, but the healthcare industry has the richest source of information on patients through the power of claims data.
Common use cases of claims data within the biopharma industry are to generate evidence for emerging therapies, disease and market landscape assessments, patient journeys, and economic value stories related to patient access and health outcomes. It’s also used to measure market performance of a treatment and to stand up commercial programs to assist patients in getting access to treatments and promotional activities for relevant key stakeholders, such as treating physicians and specialists.
Many of the emerging applications of de-identified claims data are found in the integration with other data streams, such as lab, genomic, consumer, wearable, and exposure data, among others. An example of how this is creating innovative use cases for biopharma is with measuring ROI for digital promotion campaigns. By linking exposure metrics to target audiences within real-world data, companies can more effectively measure their marketing spend by prospectively looking at the impact of the campaigns on capturing new patients and keeping patients on therapy for longer.
Integration also allows biopharma to “fill in the gaps” of the patient journey and, as such, allows for more features to be leveraged in machine learning models to identify undiagnosed patients, specifically those with rare diseases, as well as drive high-value targeting models and other predictive analytics.
Speaking of ‘filling the gaps’ and creating a longitudinal view of the patient, are there certain types of data that are particularly powerful for fulfilling those applications when linked with claims data?
Cliff: Healthcare data is so rich at the moment with the growth in precision medicine, digital therapeutics, and wearable devices — when coupled with the wealth of information available in lab / genetic testing data and unstructured EMR, claims data can now provide a much broader picture of the patient’s diagnostic and treatment pathway, as well as assessing health outcomes. Having this enhanced view will enrich longitudinal patient studies that will evolve the way biopharma is demonstrating value to key stakeholders in meeting unmet clinical needs, increasing market access to needed treatments, and improving health outcomes.
Can you provide real-world examples of how Clarivate's connected, de-identified claims data has contributed to advancing our understanding of health and disease?
Bob: Recently, a global pharma company was seeking to understand the epidemiology of disease and treatment patterns for patients with a chronic autoimmune neuromuscular disease that causes weakness in the skeletal muscles, which are responsible for breathing and moving parts of the body, including the arms, legs, facial muscles, and others.
The primary objective of this study was to leverage RWD to refine the literature estimates of the indication prevalence (37K — 112K) and provide insight into drug treatment patterns.
Our team helped the client in understanding the overall diagnosed prevalence in the U.S., by age, gender, subtypes, capturing annual diagnosed incidence, and treatment patterns by class and line of therapy.
Read more about the way Clarivate Real-World Data fuels research breakthroughs: Peer-reviewed publication highlights featuring Real-World Data - Clarivate.
The Constraints and Challenges with Claims Data
Bringing together data from multiple sources comes with its own set of challenges. How does Clarivate ensure the quality and consistency of data across different sources and healthcare systems, given the inherent heterogeneity in claims data?
Bob: There are a few processes we execute to ensure the quality and consistency of data across multiple sources:
- Patient mastering that allows for common patients to be linked across sources and types of data and enforces patient demographic integrity across all linked records.
- Extensive QA from landing through to analytic read repositories. Exceptions are flagged and, if significant, will result in the record(s) being suspended for further follow-up before promoting to an analytic repository (e.g., invalid standard medical code).
- Data received is normalized and standardized into a common model that is appropriate for the type of data.
All three processes are critical to ensuring that data blended from multiple sources is ready for analytic use. However, the QA suspense process is particularly effective in that it allows us to over-audit up front and then add back records that are found to be or become valid (e.g. invalid NDC due to lagged reference data).
How do you address privacy concerns surrounding the use of de-identified open claims data? Are there specific measures in place to ensure patient privacy and data security throughout the research process?
Bob: Clarivate only receives de-identified data from our data sources, and our data sets are regularly reviewed by an independent HIPAA Statistical Expert using the expert determination method. We build redaction rules and execute automatic ongoing redactions based on expert recommendations in order to ensure patient privacy is preserved in compliance with HIPAA standards.
If any data is added or removed from the core analytic dataset or if the core data is to be combined with any other data, then an updated Statistical Expert determination is performed and redaction rules are adjusted.
For data security, we maintain our data per industry standard best practices such as maintaining data encrypted at rest and enhanced user access and challenge controls.
The Future of Claims Data
A 2021 study used machine learning (ML) on claims data to predict hospital readmissions, improving patient care management. How do you think the growth of AI/ML will have an impact on research using claims data?
Bob: The accuracy and precision of ML will improve not only as models evolve, but as data lakes that drive the models become more robust and actionable. Broader integration of longitudinal claims data with various data streams will increase the availability and diversity of model features, thus enhancing the capabilities of ML approaches. This will be critical in research related to finding and assessing rare-disease patients, especially those with no diagnosis coding and complex diagnostic criteria — among a plethora of other use cases.
By applying RWD-driven machine learning algorithms, early detection, differential diagnosis, and risk stratification can facilitate and catalyze time to diagnosis. As an example, one of our clients had an algorithm that used EHR data to find potential patients with a specific rare disease but struggled with lower accuracy, missing many potential patients Our analytics team leveraged RWD products by inputting symptoms, diagnoses, procedures, and treatments from EHR and claims data within a five-year period into ML algorithms. Across the six ML models, accuracy ranged from 75% to 80%, including the validation test. Our client now has the ML-based algorithm that effectively identifies suspect patients with this rare disease, with the goal of ultimately reducing rates of delayed or missed diagnosis.
Provided the technology is used responsibly, AI and ML will have a significant positive impact as claims data is integrated more extensively into precision medicine applications. Moreover, it is crucial to consider the emerging regulations around AI/ML that will provide important protections to ensure that the excitement of the technological capability does not preclude responsible use and management. As the regulatory landscape evolves, the responsible integration of AI and ML in healthcare will foster advancements in regimen efficacy analytics by further enriching claims datasets with patient data from diverse modalities and devices such as Electronic Health Records (EHR), wearables, and other sources. This evolution will facilitate a migration from RWD to RWE.
Thanks for the interview. Any recommendations for our readers if they want to learn more?
- About Clarivate Real-World Data Product: Real-World Data - Clarivate
- How Clarivate expert analytics team solves challenges in using RWD: The challenges of working with real world data and key ways to overcome them - Clarivate
- About Clarivate forLife Sciences and Healthcare:Life Sciences and Healthcare - Clarivate