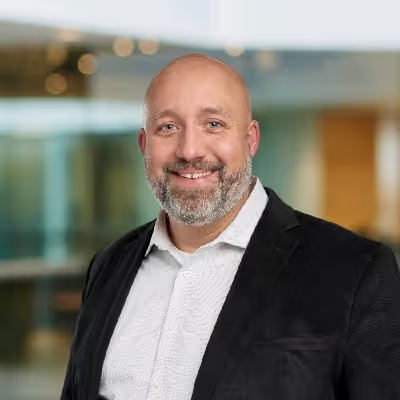
In our Ecosystem Explorer Series, we interview leaders from organizations who are advancing access to health data. Today’s interview is with Michael Swartzbaugh, Sr. Director of Product, Real-World Data and Innovation at TriNetX.
Michael Swartzbaugh, BS, is a Sr. Director of Product at TriNetX and is part of its Data & Analytics Solutions group. For the past 2 years, Michael has owned TriNetX’s linking program, Linked, focusing on expanding data beyond electronic health records (EHR), defining its product and features, and evaluating third-party data sources to support and define the strategic inclusion of other data to complement TriNetX’s industry-leading breadth and depth of EHR data. Prior to joining TriNetX, Michael spent 20 years at Cardinal Health focused on R&D, Product, and Delivery for Specialty and Payer Product Offerings.
TriNetX is the global health research network that connects the world of drug discovery and development from pharmaceutical company to study site, and investigator to patient by sharing real-world data to make clinical and observational research easier and more efficient. TriNetX combines real-time access to longitudinal clinical data with state-of-the-art analytics to optimize protocol design and feasibility, site selection, patient recruitment, and enable discoveries through the generation of real-world evidence. The TriNetX platform is HIPAA and GDPR compliant.
Introduction to electronic health record (EHR) data
Michael, thanks for being here today! Let’s start with an overview: Can you explain what EHR data is and why it's significant for healthcare research?
Electronic Health Record (EHR) data refers to the digital version of a patient's medical history, including information about their diagnoses, medications, treatment plans, immunization dates, allergies, radiology images, clinical notes, and laboratory test results. The significance of EHR data for healthcare research lies in its potential to improve patient care, streamline healthcare processes, and contribute to medical advancements.
How is TriNetX involved with EHR data? How does it fit in with your company’s mission?
TriNetX is a global health research network that focuses on connecting healthcare organizations, researchers, andLife Sciences companies. It leverages electronic health record (EHR) data to create a collaborative platform for clinical research.
Bringing together patient data from a variety of sources comes with many challenges, privacy being a primary concern. How do you source EHR data while maintaining patient privacy?
TriNetX uses a patented federated network approach to source EHR data. The platform adheres to strict privacy and security protocols to protect patient information through comprehensive data use agreements, anonymization processes, and localized regulatory requirements.
The value and applications of EHR data
What are some of the common research use cases involving EHR data? Any novel or emerging use cases that you think are noteworthy?
Common use cases that require EHR data go beyond what is commonly found in other large claim sources. Claims are built for billing purposes - procedures, diagnosis, and prescriptions for a patient encounter. EHR data’s strengths are the vitals, labs, and demographics (race and ethnicity), that are not readily available within claims data. The ‘freshness’ of EHR data also allows researchers to see the use of the drug within weeks or months of entry into the market.
Any drug that requires lab results or vital signs would benefit from EHR data. For example, a very common illness in the US is hypertension or high blood pressure. When performing a study, it is typically not enough to know if they are taking the drug, but what are the outcomes. Is the patient’s blood pressure being maintained? What is their blood pressure? How many milligrams are being prescribed? Is there a reason for increasing the dose? These types of questions can only be answered with EHR data.
Some common use cases for EHR data include:
- Treatment Patterns/Pathways — What new treatments are being prescribed for the patient?
- Treatment Response — What was the result or response of that drug or treatment — lab results or vital signs?
- Treatment Duration — How long has the patient been on a particular drug?
- Baseline Disease Severity — What are the baseline characteristics of this particular disease?
- Disease Progression — How did this disease progress amongst a larger population? Did they experience the same effects or outcomes?
- Adverse Clinical Events — Did this drug or treatment cause an adverse event?
Additional use cases when linked with mortality and claims data:
- Medication Adherence & Persistence — Is the patient filling and refilling their medications? If there was a change in prescribing patterns, why?
- Patient Survivability — What was the outcome for the patient?
With the use of tokenization becoming more available and accepted within the US, use cases that can be answered by combining multiple data sources and enriching the EHR data to create unique data sets for research purposes are becoming more prevalent which will, hopefully, lead to even better research and outcomes.
You mentioned the benefits of linking mortality and claims data with EHR data. Are there particularly powerful combinations of different data types that reveal insights into patient or population health?
For Real World Evidence and Health Economics and Outcomes Research, epidemiologists seek to combine the depth of EHR data with closed claims and mortality data for patient journey and longitudinal analysis. The closed claims data complements the EHR data (labs, vitals, diversity, diagnosis, procedures, and medication orders) by adding the patient payer enrollment details as well as the billable diagnosis and procedures for the patient records. Mortality data will be combined to answer questions regarding patient outcomes and survivability.
In addition to these structured data sources, access to unstructured data like clinical notes add additional information not readily available through the structured EHR data (Example: Oncology Staging TNM: T - Tumor Size, N — Lymph Nodes, M — Metastasized).
Open Claims data is relevant for timely transactional healthcare data covering large populations of patients when needing to perform market access and other larger population health research. However, it is limited in not having the complete patient journey or longitudinal data as Closed Claims.
Lastly, there are a broad range of unique data types emerging that we seek to combine with our EHR data for unique custom linked datasets that are fit-for-purpose for a particular disease or indication. For example, Type 1 Diabetes patients that need to have glucose levels monitored with a continuous glucose monitor. It is now possible, with the use of tokenization, to create these unique data sets by combining the medication orders and lab results present in the EHR data with device data captured in continuous glucose monitors.
That's quite a range of applications. Has TriNetX data powered some of these use cases? Can you share a particular case where connected EHR data contributed to a research breakthrough or improved patient care?
TriNetX EHR data is continually being used to help researchers by supplying real world data that can be used to better patient outcomes. Since 2018, TriNetX has been cited more than 1,200 times in publications, research papers, or posters.
A particular case that impacts a large portion of the US is obesity. There is a US obesity epidemic: 70% of American adults are obese or overweight. Treatments that can be used to help lose weight or control weight are posted all over any social media outlet. You will see different supplements, workout commercials, etc. that promote weight loss.
In 2021, the FDA approved Wegovy® (semaglutide injection — glucagon-like peptide 1 receptor (GLP1R)) for chronic weight management. This was the first such approval since 2014. This semaglutide is also known as Ozempic® for treating Type 2 diabetes. However, recent concerns were raised that the use of Wegovy®/Ozempic® would increase suicidal ideations.
The connected TriNetX EHR Federated Network and platform allowed for researching over 100 million patient records to assess the potential risk. The results published in Nature Medicine earlier this month indicating that they did not increase the risk of first-time and recurrent suicidal ideation. This is just one of many examples of the invaluable power of connected EHR data to save and improve lives.
The challenges of working with EHR data
It's commonly cited that 80% of health data is unstructured, including EHR free-text fields and clinical notes, and transforming unstructured data into structured data to enable linkage and analytics can be quite challenging. How do you approach the problem of unstructured data?
Since 2018, TriNetX has been actively engaged in annotating a range of clinical data, including diagnoses, medications, and laboratory results, extracted from clinical notes. Our approach involves resolving these annotations to established standard reference terminologies: ICD10-CM for diagnoses, RxNorm for medications, and LOINC for lab tests. This annotated data is then integrated back into our platform, complementing the structured data already available. We employ sophisticated NLP (Natural Language Processing) tooling that is particularly adept at executing complex tasks like identifying familial relationships and recognizing negations, features that are frequently encountered in clinical notes.
Does variability of data sources, formats, and entry protocols introduce inconsistencies that need to be resolved? How many different EHR systems and formats does TriNetX have to tackle data standardization for?
EHR data can be difficult to cleanse, normalize, and derive. Harmonizing EHR data at scale is a crucial part of being able to query EHRs across a federated network to create a common ontology. Our standardized terminology is the backbone of our TriNetX offering. It is based on reference terminologies for Demographics (Race, Ethnicity, Language, Vital Status), Diagnosis (ICD-10-CM, ICD-9-CM), Oncology (ICD-O), Procedures (ICD-10-PCS, CPT, HCPCS, SNOMED), Medications (RXNorm), and Labs (LOINC) that maps disparate coding systems to query against each EHRs local coding system. TriNetX supports more than 40 different EHR systems and 15 or more different integration models for over 200 Healthcare organizations.
Since the passage of the HITECH Act in 2009, provider adoption of EHRs has skyrocketed to above 90%. Has the availability of de-identified EHR data followed suit, or is access to EHR data still an obstacle for researchers?
EHR data at scale is still a challenge. TriNetX was founded to, and then did create, the largest federated network of EHR data for purposes of research, protocol design, and site identification for clinical trials research. Our network’s architecture is privacy-preserving. Currently, TriNetX is a Global Network in 35 countries with 216 HCOs with close to 300M patients. It has been amazing to see the contributors to this network that are interested in providing data for furthering research and improving patient outcomes. It has further been enriched by adding third-party data and on-platform advanced analytic offerings that reduce the burden of needing to take clinical data and ingest it into local data warehouses to perform research.
However, outside of TriNetX, EHR data at scale largely isn’t present. Other larger data aggregators started with Claims (either Open or Closed) as their foundation. TriNetX focused on EHR first with a desire to complement EHR data by linking in other clinical data sources of interest.
Other areas where EHR data is present are Health Information Exchanges (HIEs). They promised to combine the many sources of clinical data to have a central location for sharing patient information securely. However, these are also still limited in use.
EHRs are still very siloed within their organizations or larger Integrated Delivery Networks (IDNs). EHR-based research continues at academic medical centers and other institutions but may be limited to their own EHR data with an average of 1 to 2 million patient lives.
Beyond the lack of industry standardization and data silos, are there any other notable challenges researchers face with accessing or utilizing EHR data?
TriNetX has tried to remove barriers of accessing EHR data for research, whether that be allowing for complex protocols and queries, on-platform analytics, linking to other external data sources, or even having a data science platform for use with common tools like R, Python, and SQL.
However, outside of academic EHRs that will have access to local data, epidemiologists have been traditionally trained on claims data as the primary source of data for research needs. Depending on the epi topic, EHR data in the form of primary data collection (surveys and chart pulls) and EMR data may be incorporated into the training programs but is still limited.
So, there are three areas I would indicate as challenges for researchers:
- Familiarity with EHR data and what value it provides compared to claims data is always an area of growth and education.
- Harmonizing EHR data across sources and/or combining with other claims sources continues to be a challenge.
- Quality of real-world data and how to cleanse it for immediate research needs.
The future of EHR data
In the wake of the COVID-19 pandemic, much has been said about the need for near-real-time integration of EHR data for public health research. What do you see as the biggest areas of opportunity for EHR data in the coming years?
Structured EHR data that has been harmonized to perform research has been solved. Expanding beyond that to create models based on physician notes and placing them into structured fields, unlocking imaging results, and diving deeper into genomics and specialized testing to help oncology research are all opportunities within the coming years. Some of this has definitely started already but is very early on in its development of what can truly be done.
What can be done with large language models, like BERT, to help complement EHR data to try to make access, querying, or understanding what is available will be interesting to see as time goes on.
LLMs and AI/ML applications in healthcare research are certainly promising. Are there any promising technological innovations that you think will improve access to or utility of EHR data?
There are some interesting new products that fall into a few categories: reducing complexity of sharing or assessing data, global data access, and lastly the effects of NLP and models at scale.
In terms of sharing and accessing data, there are a few products seeking to reduce the time it takes to assess custom linked data sets, reduce the time for feasibility or answer questions regarding “is this data fit for my purpose?”, all while needing to ensure governance, compliance, and patient privacy is preserved.
It will be interesting to see how these evolve over time as they work through privacy and access concerns.
Another area of innovation is global data access. Expanding upon US data and being able to unlock ex-US data that is still GDPR compliant in the EU, for example, while it allows for querying in a structured, controlled, and governed way to ensure privacy compliance is of interest.
Lastly, NLP at Scale is, as mentioned above, still in its infancy of utilizing it to take unstructured data and place in structured, query-able data at scale. To unlock all of the benefits of EHR data, programs that support unstructured data will be differentiated in the next few years.
Thanks for the interview! Do you have any recommended resources for readers who want to learn more about EHR data or TriNetX?
To learn more about TriNetX, visit www.TriNetX.com. I recommend browsing the publications section to learn more about the real-world impact EHR data has on health research.