In our Data & Analytics Thought Leader Series, Datavant’s Head of Data Strategy, Su Huang, interviews leaders who are responsible for defining the teams and processes for managing data and advancing data-driven use cases at their organization. Today’s interview is with Jane Urban, Senior Director of Commercial Data Management and Strategy at Takeda Pharmaceuticals.
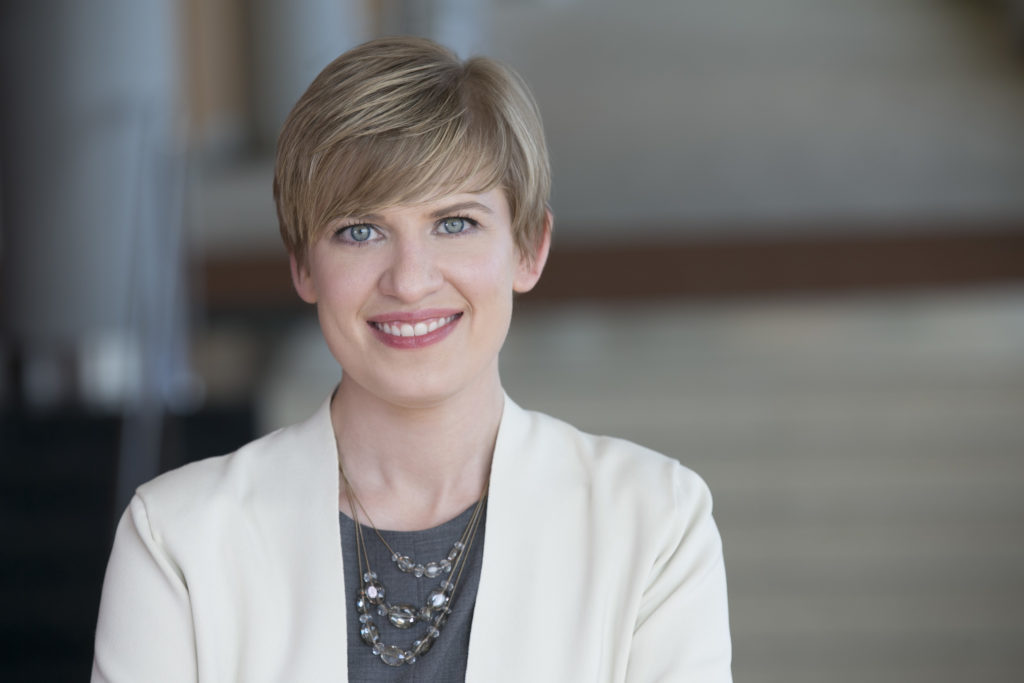
Jane’s career has spanned biomedical engineering, economics and now data science and strategy at Takeda. Over her 15+ year career, Jane has held roles in data analytics, market research, digital marketing and consulting. At every step, a deep understanding of data, analytics and the white space opportunities they present has been the key to her success. At Takeda, Jane has built a team and infrastructure to accelerate the adoption of data and analytics across the organization, spanning commercial and clinical development. Jane has also been involved in multiple integrations of data systems and processes due to mergers and acquisitions.
Su: Jane, welcome to this interview series! Throughout your career, data has been a common theme. Over the past few years, you’ve been focused on building a data and analytics discipline at Takeda. Can you describe the before and after of data management at Takeda?
Jane: The commercial data management strategy function started in 2020 and was born out of the thesis that we have incredible data assets at our disposal, but we’re not leveraging them fully. “How might we create more value from the data we already have?” was the overarching theme. That gets to the use cases that are so relevant in healthcare: How do we engage with physicians and patients better, faster and in more accurate, useful ways? How do we help patients who are already on our therapies to stay on them? Where are the therapy decisions being made? Data can really facilitate all of those things. There’s a lot that needs to be built before we can effectively answer those questions.
One of the ways I describe our team today is this idea of us being former chefs turned farmers. We’ve been in the kitchen and we know how to cook some really great meals, but we are now looking upstream to figure out how to get the absolute best raw ingredients for even better analytical techniques. So we’re off on the farm, trying to grow the best data we can. That requires a certain set of people who have a background in data analytics. Our team is part of the larger commercial operations organization, where we are forging new partnerships with our analytics colleagues who focus on each therapeutic area.
Su: I love the chef turned farmer analogy. I’d like to explore the impact of M&A and integrations on data infrastructure. You have been involved in integrating Shire’s acquisition of Baxalta and then integrating Shire into Takeda. When you’re thinking about bringing together multiple different organizations and the data assets they have, what were some of the key challenges during integration? How did you create a cohesive infrastructure spanning different business units?
Jane: This is always a challenging thing to do. You have some legacy data systems that are quite old and some that are modernized. There’s a mix. We tried to simplify the decision set for the integration of different capabilities. Shire and Takeda are both sizable companies with some mature capabilities, so it became a triage exercise: Are some capabilities so similar that we just pick one and not use the other one? Other times, there were good things about each, so we wanted to enmesh the best of both. Then there were a few areas where neither was great, so that became an innovation space where we could build something new that would be better than either company had prior to the acquisition.
I will say that the net new tended toward the data space such as master data management, because neither company was happy with its capabilities in data. There were challenges with our data infrastructure, accessing data, using data, and finding data. These aspects do not exist in a vacuum, so we have to partner with all areas of operations, analytics, and business strategy to ensure the way we are integrating is fit-for-purpose to the overall goals of the US business.
Another area is around how we catalog and keep track of all the data assets that we have. We used a data cataloging tool to bring everything we purchased all in one place. That helped us eliminate duplicative purchases and it also allowed for people to “go shopping” internally before they started to go shopping externally for data assets.
Su: Since Takeda is an international company, how does your team work with ex-US teams on data and analytics initiatives? Do you have established processes to enable collaboration? Are you able to access data sets and data science resources on a global basis? I’d love to learn more about that.
Jane: This is an area where there’s a bit of a challenge with legal requirements like the GDPR. That precludes us from doing certain things that we could do technically, but we cannot do from a legal perspective. We have a data privacy function within my team that keeps us on the straight and narrow around what we can and cannot do. We have both a data privacy person and someone focused on compliance of patient-level data. My team also works with global privacy groups. We have a global data council that meets on a regular basis and shares best practices. So even if we can’t share the data sets because of a firewall by country, we can talk about what we’re trying to do and how we’ve done it and even get into some of the details around the techniques and algorithms that we’re using.
Su: What technologies do you use to address privacy and compliance? There’s growing volumes of data available today, such as genomics data. How can pharma companies leverage that data without jeopardizing patient privacy?
Jane: We use a lot of different techniques around aggregation and tokenization to make sure we’re keeping privacy at the forefront as we establish our data sources. We leverage tools to connect data through tokenization, which keeps the data anonymized and private, but also gives us this ability to maximize the connectivity of the data. When you’re putting together lab data, claims data, specialty pharmacy data, tokenized and anonymized data allows us to unlock things that maybe we couldn’t have any other way. We don’t need to know the individual but we do want to know everything we can about a given person who has received all these different treatments in different care settings. We want to know where they’ve been and what they’re dealing with in their healthcare journey. A lot of the disease states we work in are rare and chronic – they are diseases that patients will deal with potentially their entire lives. So, the better we can use this technology to solve that problem, the more it helps patients.
Su: Are there diseases besides rare diseases where real world data has high impact today, or could have high impact in the future?
Jane: Real world data ultimately should be part of the solution for every disease state, but there are some areas where it makes more sense today. Certainly oncology has always been way ahead in terms of the value of real world evidence to see which combinations and permutations of treatment work better. Next is rare diseases where we really have to accelerate diagnosis first, and then deal with treatment. Real world evidence can help us see patterns that would be harder to see any other way. In the rare disease space, although each disease is small, there are a lot of rare diseases collectively so there’s an opportunity to look across them and hopefully diagnose patients faster and help them find a treatment that makes sense.
Su: You have described your journey in building a data function as going beyond using data for commercial use cases. You are partnering with R&D and finding common data assets that you can leverage. Can you tell me about the process of collaborating on these kinds of data initiatives with other parts of the organization?
Jane: This is something I’m really excited about. It’s been a really great area, beyond the original scope of commercial data management and strategy. There’s a big change in mindset that’s happening across the organization. That is what is really unlocking the power of collaboration around data – this idea of trying to look for opportunities to share rather than not share.
The other is the algorithms. We’ve been experimenting with how to build agnostic algorithms for predicting the potential for a given patient to look like a patient that actually has the rare disease we’re studying. That’s been applicable to both clinical trial recruitment, for example, as well as post marketing analytics. So in spaces where we have both candidate products and market products, there’s an opportunity to share algorithms that we’ve built. Even if we don’t share the intention of the algorithm, we can share the idea of the prediction model.
Being able to share the same algorithm between commercial and medical teams has been a really great opportunity for talent to collaborate with the appropriate firewalls of not influencing each other.
In terms of data science, it’s buzzy to talk about algorithms and AI/ML. But people are starting to understand the value that a great data engineer can unlock, so we’ve been pairing our data engineers with our data scientists. That’s where I think some of the real magic is happening. A data engineer and a scientist working together brings focus for the data engineer because it can be overwhelming to clean up a massive pile of data. Data engineers struggle to know where they should even start. The scientist gives them context to focus on a specific area of the data. For the scientists, they’re struggling with how to write the correct query. The data engineer can help them with that, and also create a repeatable solution to operationalize the process rather than just a one-off extraction of data for a single project.
A great example of this is seeing our marketing leads and franchise heads who went from an initial set of questions to asking even more complicated and nuanced ones. The first question was often: “Are there patients that we’re missing?” Now it’s become: “Why and where do they come from? What’s happening next? How do we predict more of that?” So, a nice flywheel effect is starting to happen in the therapeutic areas where we’ve started first. In turn, that’s piquing the interest of other franchise hands and other marketing teams who are saying, “Hold on, I want some of this too!”
Su: Zooming out, where do you think most pharma organizations are in the maturity scale for using real world data (1 = nascent; 5 = mature)? What does the industry need to get to the next phase?
Jane: I think we lag pretty significantly behind the consumer space where there are fewer restrictions in terms of what’s allowed. I’ve described it as a bullet train flying down the tracks. You’ve got Google and Amazon in the front car, blazing the trail. Pharma is at the very back. We’re using that train to figure it out and accelerate our growth. At least at Takeda, I would say we’re in the mid 2 to 3 range of the maturity scale overall, with some areas that are very mature and some that are still getting started. We’re not completely nascent, but we still have a long way to go. There are a few pharma companies that are ahead of Takeda for sure, but the nature of the data we work with and the very real privacy concerns will tend to keep us in the middle to later stages of innovation.
To get further ahead, I’d like to see more emphasis on reusing data, and of using a product-based, software development model. As you develop something that is a widget or a tool that has an input and an output, can you then reuse that in other applications? If we’re trying to predict the likelihood that a patient has a rare disease, how do we modularize that so certain components can be reused? Another side of that is documenting the data’s origin and the history of building the infrastructure in a systematic way. We sometimes tend to reclean data due to lack of awareness that it’s already been cleaned.
Su: Thinking about pharma’s collaboration with payers, how important do you believe enhanced collaboration with payers, both commercial and government is for the success of pharma companies moving forward? Why?
Jane: This is an area that’s continuing to grow and where there’s a need for collaboration. We are leveraging claims data as a key source for both real world evidence analytical work as well as payer engagement with physicians and patients. How can we better understand where there are access barriers? What limitation on choice do patients and physicians face when looking at treatment options? Is there an algorithm or paradigm that makes sense from a science perspective to answer those questions? Or is it an opportunity to bring value from a patient lens to the payers’ doorsteps so they understand why physicians and patients want that access? When products launch, we can get better at understanding how the payer landscape is evolving and reacting to a new product entering the market. We can also get better at predicting where there could be potential issues in the future.
Su: Last question — If you had no constraints and could accelerate one area of what you are overseeing at Takeda, what would that one area of investment be?
Jane: I would try to look at how we collaborate. How do we build innovative things using data as the core? Perhaps implement multidisciplinary pods like what you see at software companies. We can have people coming from different mindsets, different backgrounds: medical doctors, data engineers, data scientists, different commercial folks coming together in a “one pizza” small group. Ultimately, that’s where we have to go as a pharma organization. It sounds a little far-fetched right now, but my hunch is that the way we solve data problems will ultimately look more and more like developing software.
Su: Thank you so much, Jane, for being part of this Data & Analytics Thought Leader series!
Disclaimer: The opinions expressed in this interview are solely those of the presenter and not necessarily those of Takeda. Takeda does not guarantee the accuracy or reliability of the information provided herein.
If you would like to learn more about this series, please email Su Huang at su@datavant.com.