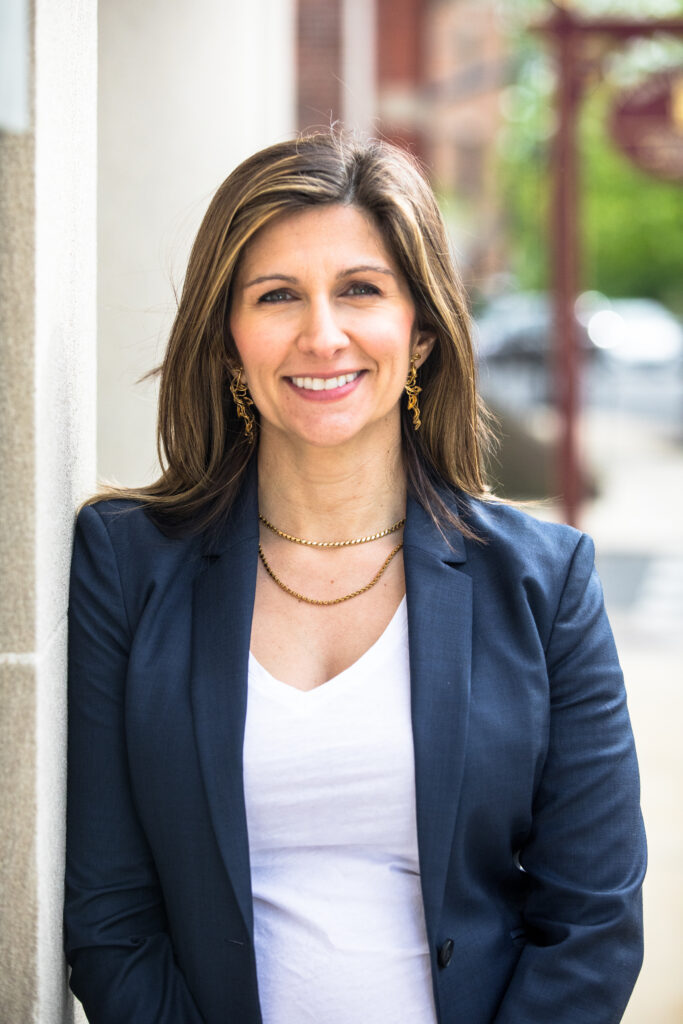
In our Ecosystem Explorer Series, we interview leaders from organizations who are advancing access to health data. Today’s interview dives into the world of multimodal data with Miruna Sasu, CEO at COTA.
Miruna Sasu is the Chief Executive Officer at COTA. She has held leadership positions at Johnson & Johnson and Bristol Myers Squibb where she revolutionized company-wide digital innovation and advanced analytics across enterprise drug portfolios from drug discovery to value access and post-marketing.
Miruna holds a PhD in biology and statistics from Penn State University and an MBA from Temple University.
COTA was founded in 2011 by doctors, engineers, and data scientists to create clarity from fragmented and often inaccessible real-world data (RWD). By using our proprietary technology, advanced analytics and deep expertise to organize complex data, we provide a comprehensive picture of cancer that can be used to advance care and research. We believe that everyone touched by cancer deserves a clear path to care. Together, we can make that vision a reality.
Introduction to multimodal data
Miruna, welcome to our Ecosystem Explorer interview series! For readers unfamiliar with multimodal data, let’s start with the basics. What is multimodal data, and why is it important to healthcare researchers?
Multimodal data, or multisource data, is data that has its origins in different areas of the healthcare continuum. These data sources may include EHR data, claims, laboratory and pharmacy records, molecular profiles, medical device data, and patient-reported data on surveys, questionnaires, or even social media.
Multimodal data is important because humans are multidimensional. When we capture different data types that reveal different aspects of a person’s healthcare experience, it results in a fuller and more accurate picture.
What is COTA’s connection with multimodal data? How does it fit in with your company’s mission?
COTA was founded to make sense of fragmented EHR data, in order to give a longitudinal view of the patient journey. Doctors and drug developers have so much data at their fingertips to help them fight cancer. They have the potential to query information on millions of clinical outcomes to find the best course of treatment for a patient, or identify the groups that’ll benefit most from a new medicine. Our medical records – after being de-identified to protect our privacy – hold the keys to countless discoveries about how cancer is diagnosed and treated. So what’s holding us back from using them right now to cure someone’s cancer?
If we’re serious about helping patients with cancer live longer, healthier, cancer-free lives, we need to get serious about improving the quality of cancer data. This means making sure our cancer datasets represent diverse groups of people and treatment settings and data sources. When we use multimodal data, we can portray patients’ journeys completely and accurately, and include the data points that matter most to answer tough cancer questions.
When it comes to answering those tough cancer questions, are there special applications or benefits of multimodal data to oncology research?
Multisource clinicogenomics data can assist with monitoring the long-term effects of a therapy as part of Phase 4 safety studies, identify any long-term secondary impacts of the product, and help to appropriately position the product in the market to ensure maximum benefit to both the developer and to patients.
These approaches are already producing benefits for patients. For example, many people cannot tolerate statin therapy for high cholesterol, despite the fact that statins are the gold standard treatment for this condition.
When researchers examined genetic data to gain understanding of the mechanism of action of high cholesterol, they found that certain mutations (proprotein convertase subtilisin kexin type 9) played a role in development of the disease. They used these insights to develop an alternate therapy using monoclonal antibodies that target the mutation. This led to the launch of two groundbreaking drugs, evolocumab, and alirocumab, that provide suitable alternatives to statin therapy.
This is a classic example of genetic evidence playing a crucial role in drug approval. With the rich clinicogenomic RWD that is available today, we are likely to see more success stories like this one alongside reduced costs and faster development of life-saving drugs for patients.
That’s a great example. Are there other real-world applications that come to mind that illustrate how multimodal data has been used to improve cancer diagnosis, treatment, or research outcomes?
While cancer affects roughly the same number of women as men, similar numbers of Black and White Americans, and people living in urban and rural parts of the country, a group of factors known as social drivers of health (SDoH) may mean one person’s cancer outcomes are worse than their neighbor’s. SDoH includes things like where a person lives, the level of education they’ve received, and how much money they make – things that, though not directly related to medicine, can impact health. If you live 45 minutes from the nearest medical center and don’t have a job that offers paid time off for doctor’s appointments, it’s unlikely you’ll be able to participate in clinical trials for novel treatments. It can be a matter of getting a life-changing treatment or not.
Multimodal datasets reflect the real lives of the real patients we treat. When data used to answer important questions about cancer represent only a small sliver of the population, the results may be skewed or biased. That’s not useful for drawing conclusions about how cancer affects Americans more broadly. For example, we can’t use data from affluent, majority-white patients treated at an academic medical center in a big city to understand the ways people of color in a low-income community are treated in local hospitals – the results simply wouldn’t match up.
The challenges with multimodal data
Collecting, curating, and connecting health data can be complex, particularly with data from multiple sources and types. What do you see as the major challenges with multimodal data?
Bringing all of this data together into a curated, fit-for-purpose dataset to support research and development can be a challenge, because these data sources weren’t originally designed to fit together neatly. Instead, researchers and analysts must consider a number of different issues when synthesizing multimodal data, including how to accurately identify a single patient via tokenization or other means.
Data scientists must carefully balance the volume of data (a higher number of data sources could create a richer, more complete portrait of an individual) with the data integrity and governance issues of merging many sources together.
The challenge becomes even more complicated as we start to explore other datasets to augment our current core sources of RWD. For example, we are increasingly integrating imaging data, personal device data, SDoH data, and patient experience data into the RWD ecosystem. These sources tend to be even “messier” than other data types due to poor standardization and high amounts of unstructured information.
How does COTA address this data messiness?
In cancer, this means working directly with oncologists and high-quality cancer data to make sure the data is accurate, useful, and well-positioned to drive better outcomes for patients. With the triad of strong tech, great data, and oncology expertise, we can make multimodal data the best it can be for giving useful cancer insights at scale.
As you know, data security and patient privacy are critical for organizations working with connected health data. Does multimodal data present unique challenges in those areas, and how do you address them?
Trust is the operative word when it comes to bringing together multiple data sources to create new assets for use in the life sciences environment. As we start looking at bringing multimodal data together for regulatory and decision-making purposes, we need to connect up these disparate silos in a compliant and secure manner.
We need to access and share de-identified data in a HIPAA-compliant manner while preserving the provenance and governance of the data. But more importantly, we need to protect the spirit of the law around patient privacy and respect the fact that these are very sensitive data elements.
By employing technologies that can identify, aggregate, and synthesize data in a trusted and neutral manner, the health system can start to unlock previously unused data to augment the traditional clinical trial and post-market surveillance ecosystem.
Innovations and opportunities
Let’s look to the future: What are the current trends or innovations with multimodal data?
Pharma can use high-quality data on real patients’ outcomes to de-risk cancer drug development. They can test hypotheses about how new drugs or molecules would affect patients in the data, before they recruit for a clinical trial, allowing drugmakers to predict which types of patients are most likely to benefit from their medicine. By replacing their control groups with external control arms, patient data replaces the non-treatment arm in a clinical study. This ensures all patients in the trial receive the novel therapy, rather than joining a trial only to represent standard of care. And when planning a clinical trial, they can combine this RWD with AI to model whether or not their trial will be able to demonstrate the true potential of their new treatment. Based on the outcome, they can decide whether to proceed with the trial as is, adjust its methodology to improve its chances of success, or scrap it altogether.
By testing hypotheses and trial feasibility virtually ahead of a full-scale clinical project, pharma teams don’t spin their wheels on efforts that aren’t likely to succeed. Instead, their resources are then better spent on research into other promising trials or medicines. And, critically, patients who participate in trials are protected from taking therapies that aren’t likely to treat their disease.
Much has been said about the advancements of AI/ML and its potential to transform healthcare, from predictive modeling and precision medicine to clinical decision support. How important is multimodal data to these applications of AI/ML?
It’s an exciting time to be in tech. Large language models and generative AI are top of mind everywhere. In healthcare, I’m increasingly inspired by our potential to elevate data science with AI, driving even faster answers for patients, doctors, and drug manufacturers.
AI will not work without high quality multimodal data. The promise for generative AI as a breakthrough tool to transform cancer therapy is immense: sophisticated algorithms to help doctors and researchers find insights that will uncover treatments to transform cancer care and help millions of people live healthy lives. So much is riding on it. The engine is ready to run. But there isn’t enough fuel to make it roar. Health data is notoriously incomplete, contains errors and bias, and is housed in many incompatible formats, as well as siloed in heavily protected and regulated systems across thousands of institutions. As much as 70% of data generated in sophisticated computer systems still needs to be qualified by human experts at great cost in time and resources. And there is already evidence that generative AI systems can amplify gaps, errors, and biases. Quality data – essential for AI’s clinical insights – isn’t produced fast enough. AI hasn’t passed the quality test.
High-quality data is the key ingredient to realizing the full potential of AI. By meshing automation and supervision of high-quality RWD, COTA is providing useful information at a scale that will begin to change the dynamics of cancer care. The average handle time of COTA’s product has decreased year-over-year by 10%. Instead of 70% of data qualified by human experts alone, COTA is moving towards a day when 70% of insights can be validated using AI with the same caliber of expert human oversight. The impact on patients will make the excitement around AI meaningful.
What do you see as the most exciting opportunities for health organizations working with multimodal data in the coming years?
As the cancer-treatment landscape becomes ever more complex and the disease is treated as chronic rather than a death sentence, oncologists and drug developers need a “Waze for cancer” to navigate the winding roads of treatment and trials. There’s work to be done to get there; the technology isn’t quite ready to give us the guidance we need to treat patients with precision and speed. The main roadblock lies in the cancer data landscape. Today, we are not processing data at a scale and speed necessary to power a Waze for cancer research. The information is housed in diverse data sources, but it must be processed. That makes it hard to draw conclusions and find the right path.
On the road to a Waze for cancer, we must first improve the quality and quantity of cancer data that powers AI models and infuses medical knowledge into cancer datasets – a big task, but not insurmountable. Thanks to leaders in cancer data, standardizing the way cancer data is recorded and working with oncologists to verify its accuracy is already underway. Part of this effort includes validating data and AI-driven conclusions in real time. Similar to how Waze users are asked to verify if one user’s report of a car accident or speed traps on a route are accurate, oncologists assess the data to make sure AI models are reaching the right conclusions.
Thanks for the interview, Miruna! Do you have any recommended resources for readers who want to learn more about multimodal data and its applications in oncology?
Follow COTA on LinkedIn to learn more about the future of multimodal data in cancer care.
This interview is part of our Ecosystem Explorer Series, in which we interview leaders from partner organizations who are improving access to health data. If you’re interested in participating in this series, send us an email at info@datavant.com.